Last weekend, on a whim, I asked GPT-4 “Please teach me how to create simple neural networks in Python using PyTorch.” I wasn’t sure how well that would go, but figured it was worth a try.
I not only learned how to do that, but found out that GPT-4, at least when it isn’t making things up, is an amazing tutor. It not only provided the relevant code, but explained how it works, helped walk me through some snags installing the required libraries, and is continuing to explain how to tweak the code to implement new features of PyTorch for more efficient network training. I can now analyze arbitrary .csv file data with neural networks. Amazing.
I had heard that, given sufficient data, a complex enough network, and enough training time, neural networks can learn the patterns underlying almost anything. So I decided to see how well it would do with the task I always start out with when learning a new language — generating images of the Mandelbrot Set.
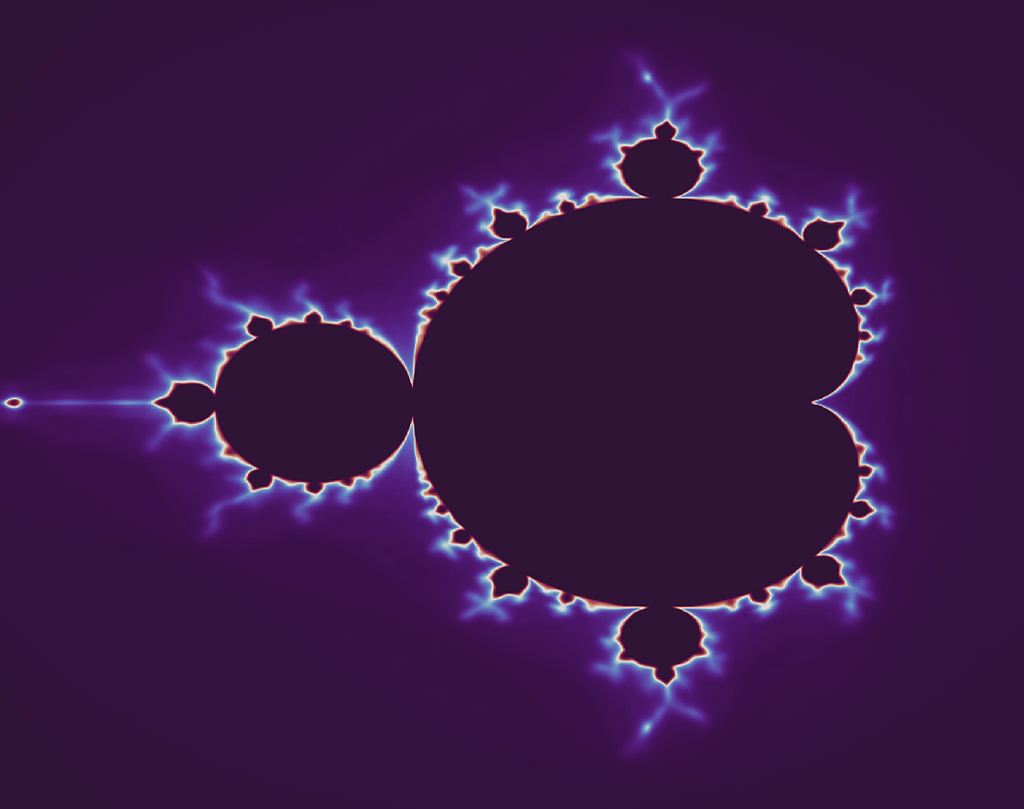
An image of the Mandelbrot Set, as imagined by a 1500-neuron network.
That’s far from the best image of the Mandelbrot Set I’ve ever created — but there’s a reason. As JFK said, we do such things “not because they are easy, but because they are hard.” The Mandelbrot Set is, after all, a literally infinitely-complex object. Keep zooming in, and there will always be more somewhat-similar-but-not-quite-identical detail.
Creating such images is traditionally done by calculating the number of iterations for each point in the image, and coloring the point accordingly. The neural-network approach I used (which to be clear is not even approximately the most efficient way to do it) does it somewhat differently. Data on millions of randomly-chosen points and their associated iteration levels is stored in a .csv file. This file is then read into memory and used as the training (and verification) dataset to train a feedforward neural network to learn what iteration levels are associated with what points. Then, when training is done, this network is used as the function to draw the Set — it is queried for each point in the image instead of doing the iteration calculations for that point.
This network doesn’t have vision. It is given a pair of numbers (representing the location in the complex plane) and outputs a single number (its guess at the iteration level). The image is somewhat unclear because it was, in effect, drawn by an “artist” who cannot see. It learned, through massively-repeated trials, what the Set looks like. Nobody “told” it, for example, that the Set is symmetrical about the X axis. It is, but the network had to figure that out for itself.
At first, the images only approximately resembled the Mandelbrot Set. But neural network design is still very much an art as well as a science (at least for now), so increasing the width and depth and switching to Kaiming initialization (to avoid the vanishing gradient problem) resulted in an image that meets my initial goal: the locations of the second-level Mandelbrot lakes are visible. The coloration at the edges even hints at the infinitely-thin “Devil’s Polymer” links that connect the mini-lakes to the main lobes.
GPT-4 still does get some things wrong. When asking it to guide me through tasks like obtaining a diamond pickaxe and then an Elytra in Minecraft (tasks that I know well), it mostly did a good job, but didn’t seem to know, for example, that hunger, Endermen, and Pillagers are not things that you have to be concerned about when playing on Peaceful mode. But even so, I was able to follow its directions to accomplish those goals.
This is a new form of intelligence, if perhaps not sentience just yet. I’ve often said that I love living in the future. It just got dramatically more futuristic.